EXECUTIVE SUMMARY
- It is estimated that more than half of occupations in the Malaysian agriculture sector have a high probability (>0.70) of becoming automated in the next one to two decades.
- Although almost every agricultural occupation has full or partial automation potential, the risk of complete job displacement is very low, as automation basically targets particular tasks rather than entire occupations.
- The tasks most susceptible to automation are those that are codifiable and routine-based, while non-routine tasks that require creativity, analytical and problem-solving skills are most resistant to automation and computerisation.
- As the nature of work changes with automation, agricultural workers will need to acquire required skills and adapt to working alongside machines. Reskilling and upskilling of workers will be critical.
- To ensure employment in the future of work in agriculture, skills development policies should focus on matching supply to current demand for skills, assisting agricultural workers adjust to change, and anticipating future skills needs.
Introduction
As the economy develops, the role of the agriculture sector as employer declines. Over time, the sector involves a declining share of the working-age population, suffers more local labour shortages and becomes more dependent on foreign workers. Between 1990 and 2020, the share of agricultural employment to the total workforce in Malaysia fell from 26% to 10.5% (Figure 1). Although the shift in economic activities from an agricultural to an industrial and services basis is the main driving force behind the decline in agricultural employment, mechanisation and technological progress is also a reason for this reduction.
Figure 1: GDP per Capita vs. Share of agricultural employment to total labour force in Malaysia, 1990-2020
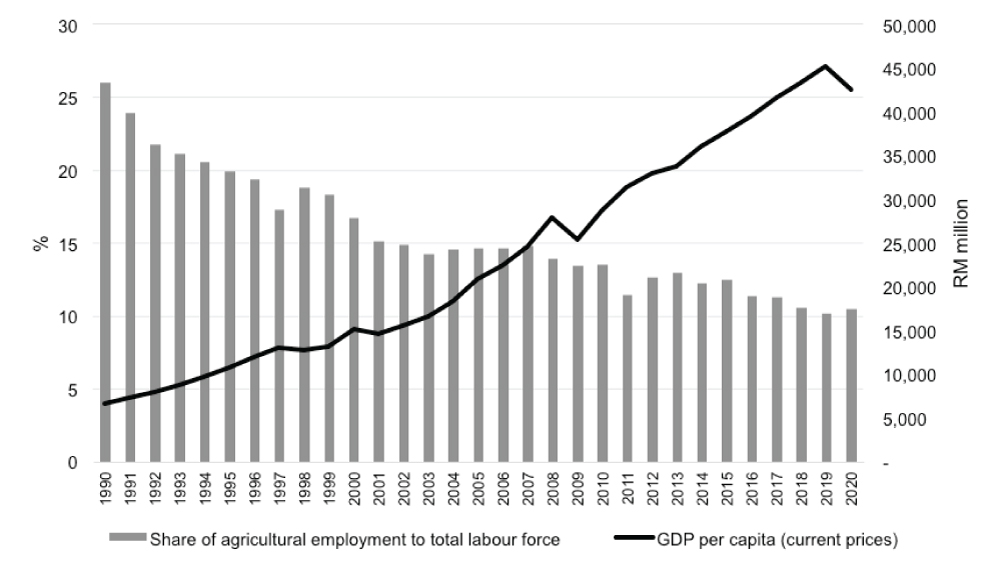
In Malaysia, agricultural mechanisation has been an ongoing trend for decades. Over the past two decades, agriculture began moving from mechanisation towards digitalisation[1]. In fact, the novel aspects of current developments in mechanisation or automations are the increased application of robotics and digital technologies for jobs that were previously only capably done by human, such as milking cows, irrigation and seeding. With pressure on food supplies likely to mount in coming decades, mainly due to population growth and climate change, applying digital technologies can boost agricultural productivity, as well as increase reliability, efficiency, and resilience of food production systems. Application of advanced technologies make agriculture more profitable while also reducing the ecological footprint of farming (e.g. by minimising the amount of pesticides and fertilizer used). Additionally, automation and digital technologies can help mitigate shortages in the agricultural labour market. At the same time, they can reduce the need for humans to do physically hard (e.g. in conditions of high heat and humidity), repetitive, and dangerous (e.g. exposure to chemicals during pesticide use) work.
Despite all of the above-mentioned advantages, as technologies rapidly advance, concerns over the future of labour increases. For instance, some scholars and policy makers have argued that automation and digitalisation might result in major job displacement, especially in labour-intensive sectors such as agriculture (Frey and Osborne, 2013; Rotz, et al., 2019). At the same time, several studies have shown that new digital technologies and automation offer various opportunities such as the emergence of new occupational profiles, the creation of more productive jobs and new earnings for workers (Autor, 2015; Bessen, 2019).
In fact, many activities that agricultural workers carry out today have the potential to be automated in the future. Yet, the effects of technological change on the labour market highly depends on the task composition of work activities. It also depends on how computers substitute for or complement workers in carrying out particular tasks, and how these tasks substitute one another.
Substitution Effects vs. Complementarity Effects
According to Autor et al. (2003), work activities can be classified into non-routine tasks (analytical or interactive) and routine tasks (cognitive or manual). A task is “routine” if it can be accomplished by machines or computers following explicit programmed rules. Many manual and cognitive tasks that agricultural workers perform, such as operating machine, driving tractors, seeding and weeding, fit this description. Since these tasks need regular repetition of an unwavering procedure, they can be fully specified with programmed instructions and performed by machines. On the other hand, “non-routine” tasks cannot be easily codified and executed by machines. They need workers with analytical and problem-solving skills as well as creativity. Hence, computers can be complementary to non-routine tasks as they can improve the productivity of workers performing these tasks. Agricultural managers and agronomists are occupations that are highly saturated with these tasks.
These therefore indicate two different effects of computer application: a substitution effect where routine work is substituted, and a complementarity effect which supports the creativity, flexibility, and complex communication of non-routine tasks.
Agricultural Occupations with High Potential for Automation
This research applied the Frey and Osborne (2013) approach[2] to categorise occupations in the Malaysian agriculture sector (farm and nonfarm agriculture-related jobs) according to their susceptibility to computerisation[3] and to estimate the probability of automation for each of them. In order to assess the automation potential for the occupations in the agriculture sector, the Malaysia Standard Classification of Occupations (MASCO) 2020 (four-digit and six-digit occupation levels) and the Standard Occupational Classification (SOC) employed in the original Frey and Osborne study were matched according to their code and title. MASCO has been developed in accordance with International Standard Classification of Occupations (ISCO). Jobs in the Malaysian agriculture sector were therefore categorised into 44 occupational groups, subjected to the data availability.
Each occupation was classified as either having low (less than 30%), medium (30% to 70%), or high (more than 70%) potential for automation based on their estimated probabilities. It should be noted that the methodology applied only provides an approximation, not a prediction, mainly due to the data limitation.
The study found that more than half of total occupations in the Malaysian agriculture sector have high potential to be automated (Table 1). It means that associated occupations are potentially automatable, perhaps over the next 10 to 20 years. Across all agricultural occupations, jobs with high capacity for automation are agricultural technicians, inspectors, crop growers and animal producers, subsistence farmers/fishermen and gatherers, fumigators, pest and weed controllers, and labourers. Most of them are jobs that mainly require routinized physical activity. Meanwhile, non-routine cognitive tasks such as agricultural managers and agricultural scientists seem less automatable.
Table 1: Occupations in the Agriculture Sector in Malaysia with Potential for Automation
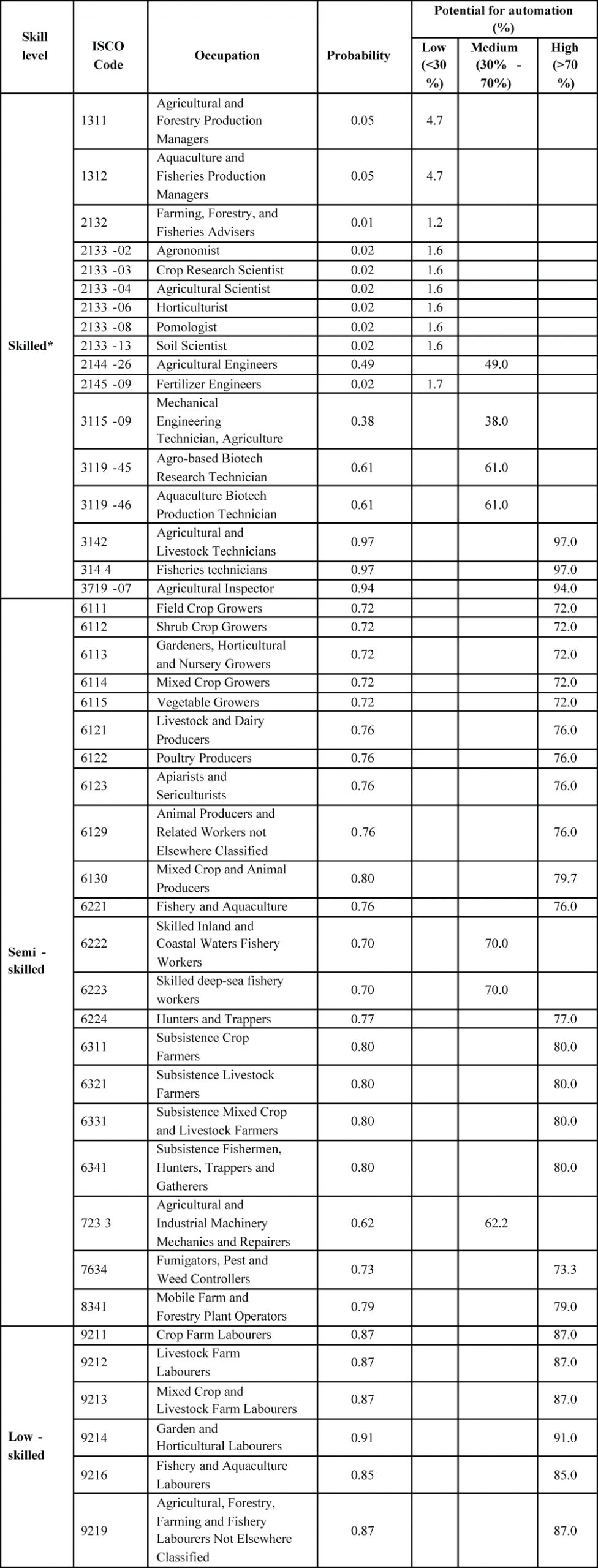
The impact of automation on agricultural workers varies across different skill levels. While very few skilled occupations can be automated almost entirely, more than 80% of middle-skilled occupations and about 100% of low-skilled occupations in the agriculture sector have potential to be fully automated. Based on the latest annual economic statistics for agriculture[4], in 2017, middle-skilled workers made up about 86% of the workforce in the Malaysian agriculture sector followed by low-skilled (8.7%) and skilled workers (5.2%). This shows that the share of occupations that could potentially experience automation remains high in this sector.
Furthermore, it is found that there is a possible overlapping between the routine and the skill content of occupations. Basically, high skilled workers tend to specialise in non-routine tasks, while more routine intensive tasks tend to need less skills (Marcolin et al., 2016). The substitutability between computers and labour is mostly driven by lower-skilled workers. By contrast, the complementarity of computers with agricultural workers is driven by the high-skilled. In fact, computerisation of some low-skill tasks can be complementary to high-skill ones. For instance, crop growers are in the high-potential category. At the same time, agricultural production managers, who rely on input from crop growers, are in the low-potential category. Therefore, automation of operations and activities done by crop growers such as preparing the soil, sowing, planting, and harvesting of crops will complement the work of production manager.
The occupations that are most resistant to automation and computerisation are those that have three characteristics, namely creative intelligence, social intelligence, and a high degree of perception and manipulation (Frey and Osborne, 2013). For instance, the low susceptibility of agricultural managers and scientists to automation is largely due to the high degree of creative intelligence they require. Hence, while it is evident that computers are entering the domains of science, there are strong complementarities between computers and labour in science and management occupations. A good example of that is the implementation of machine-learning approaches on cognitive non-routine tasks such as yield prediction, crop-quality estimation, and soil-conditions identification.
Shift Toward Higher Skills
The adoption of automated technologies is changing the nature of employment and labour demand in the agriculture sector. Employment statistics in Malaysia show that labour demand for skilled agricultural workers increased by 4.6% between 2015 and 2021, while the middle-skilled labour demand[5] grew by only 0.3% and low-skilled significantly dropped by about 34% (Department of statistics Malaysia, 2021). This is in fact the period that the Malaysian agriculture sector saw an intensive application of robotics and technologies such as drones, internet of things, and artificial intelligence. Combined with a general increase in the demand for higher-level skills, the automation of routine tasks is also the important contributor to this structural change. Adjustment costs will most likely be borne by the middle- and low-skilled workers who currently perform automatable tasks. In the long term, the shift in the occupational composition might lead to increases in inequality (e.g. wage inequality) as well as a polarisation in employment if no recovery plans for the affected labours are implemented.
Concluding Remarks and the Way Forward
Even though almost every agricultural occupation has full or partial automation potential, the risk of complete job displacement is very low. This is mainly because every occupation includes multiple types of tasks, each of which has different requirements for automation, and automation basically targets particular tasks rather than occupations themselves. It means that the nature of work will most likely be reshaped since their associated tasks become automatable. While the technical potential is an essential precondition for automation, it is not the only determinant that an activity will be automated. There are other important factors that might affect the pace and degree of automation and digitalisation in the agriculture sector. For instance, cost of technology is an important factor that could constrain its adoption. Farmers or farm owners may not adopt advanced technologies until the cost of buying and applying them at least equates the cost of labour. In a traditional industry like agriculture, convincing farmers to adopt modern technologies and apply new farming methods is another challenge that needs to be considered as well. Statistically, most farmers in Malaysia are relatively elderly, and the rate of technology adoption among them has been very low.
Skill shortage can also slow the rate of technology adoption. As the nature of work changes with automation, trained and skilled workers will have to be available to complement new advanced technologies. Agricultural workers will need to acquire required skills and adapt to working alongside machines.
A key challenge moving forward will therefore be worker retraining or reskilling not only in technological skills, but also for non-routine analytical skills involving creativity, complex information processing, and problem solving. Upskilling and retraining workers for more skill-intensive employment, not only on farms but also throughout the food supply chain, is required. To avoid the skills mismatch between labour market demand and supply in the agriculture sector, a proper labour market information system to generate, analyse and disseminate reliable occupational information is also required. More data on the type of jobs available in the agricultural labour market at the aggregate level need to be generated in order to better inform policy makers as well as help develop and target particular policy measures.
There is a need for a long-term perspective, anticipating the skills that will be required in the future, especially at the farm level, and aligning training provision with changing needs in the agricultural labour market. This applies to change in the types and levels of skills required as well as in occupational and technical areas. Taking all of these factors into account, to ensure employment in the future of work in agriculture, skills development policies should therefore focus on matching supply to current demand for skills, assisting agricultural workers adjust to change, and anticipating future skills needs.
For list of references and appendix, kindly download the document to view.
Editor: Ooi Kee Beng
Editorial Team: Sheryl Teoh, Alexander Fernandez and Nur Fitriah (Designer)
[1] Digitalisation of agriculture refers to the application of digital tools such as GPS and sensors which collect data to help farmers make more site-specific and informed management decisions (Rotz et al., 2019). Meanwhile, automation in agriculture (smart farming) refers to technology that makes farms more efficient and automates the production cycle.
[2] Frey and Osborne estimated that almost every occupation can be automated within the next two decades, except those that involve high amounts of creative intelligence, social intelligence, and perception and manipulation.
[3] Computerisation refers to job automation by means of computer-controlled equipment.
[4] Annual economic statistics 2018 Agriculture, Department of Statistics, Malaysia.
[5] Labour demand comprised of filled jobs and vacancies.
You might also like:
An Inquisition into Malaysia’s PADU Subsidy Targeting, and Beyond
Tighter Collaboration Needed in Penang’s Skills Development Infrastructure
Future-proofing Malaysia’s Health Workforce
Four Tourist Zones in Penang: Suggestions to Secure Their Future
Decentralisation is the Best Pro-Growth and Pro-Poor Economic Strategy for New Malaysia